In today’s digital age, the importance of cybersecurity cannot be overstated. As businesses increasingly rely on technology to drive growth and innovation, they also face a growing number of cyber threats. Traditional cybersecurity measures, while essential, are often reactive and can struggle to keep up with the evolving nature of cyberattacks. Enter Artificial Intelligence (AI) and Machine Learning (ML). These technologies are revolutionizing the cybersecurity landscape, offering proactive and dynamic solutions to safeguard businesses. In this article, we will delve into how AI and ML are enhancing cybersecurity and present three compelling case studies that underscore their transformative impact.
The Expanded Role of AI and ML in Cybersecurity
AI and ML stand as twin pillars in modern cybersecurity frameworks, offering a blend of versatility, efficiency, and predictive prowess. Their roles are multifaceted:
- Enhanced Threat Intelligence: AI enhances cybersecurity by integrating threat intelligence, a critical component in understanding potential cyber threats and vulnerabilities. By analysing data from various sources, AI can identify patterns and trends in cyber threats, providing businesses with an informed perspective on the potential cyber risks they face. ML contributes by continuously learning from new data, ensuring that threat intelligence is always up to date.
- Improved Accuracy in Anomaly Detection: Traditional cybersecurity measures often generate false positives, leading to wasted resources on threats that aren’t threats at all. ML algorithms are trained to distinguish between normal and potentially malicious behaviour with higher accuracy, reducing the number of false positives and ensuring that teams focus on genuine threats.
- Advanced Identity and Access Management (IAM): AI and ML are revolutionizing IAM. Biometric login methods, behaviour analysis, and continuous user verification are some aspects where AI is making significant improvements, ensuring that only legitimate users can access the systems.
- Smart Incident Response: When a cyber incident occurs, time is of the essence. AI-driven automation helps organisations respond to incidents faster and more effectively. For instance, an AI system can automatically contain a breach within minutes of detection, reducing potential damage.
- Regulatory Compliance and Risk Management: AI and ML can help businesses stay on top of the complex web of cybersecurity regulations. By analysing the regulatory environment and assessing internal processes against compliance requirements, these technologies can help businesses identify potential areas of risk.
Expanded Benefits for Businesses
The integration of AI and ML into cybersecurity strategies offers businesses a competitive edge, ensuring robust security postures and optimised operations:
- Scalability: As businesses expand, so do their networks and the potential entry points for cyberattacks. AI and ML systems can effortlessly scale alongside the business, maintaining a consistent level of security without the need for significant resource increases.
- Enhanced Data Protection: With the rise of big data, protecting sensitive information is more critical than ever. AI and ML provide advanced encryption methods and intelligent threat detection to safeguard valuable data from breaches.
- User Experience Optimization: By reducing false positives in threat detection, users face fewer disruptions, ensuring a smoother and more efficient experience. For instance, fewer false alarms in fraud detection mean legitimate transactions are less likely to be blocked.
- Strategic Resource Allocation: With AI and ML automating routine cybersecurity tasks, human resources can be redirected to more strategic initiatives. This not only optimizes costs but also allows for human intelligence to focus on areas where it’s most needed, such as decision-making and strategy development.
- Building Customer Trust: Robust cybersecurity measures foster trust among clients and partners. By demonstrating that cutting-edge technology is being used to protect sensitive data, businesses can strengthen relationships and enhance their brand image.
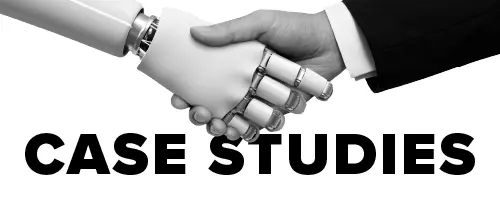
Case Study 1: Darktrace and Threat Detection
Darktrace, founded in 2013, has emerged as a pioneer in cyber defence technology, utilizing machine learning at its core. Their flagship technology, the Enterprise Immune System, is inspired by the human body’s immune system and is designed to detect and respond to cyber threats due to its self-learning capability.
Real-world Incident: In an incident involving a biotechnology company, Darktrace’s technology was instrumental in identifying and thwarting an insider threat. The system flagged unusual data transfer on the company’s network, which was traced back to an employee who was transmitting sensitive data outside the network. The employee, who was resigning, attempted to take proprietary data with them. Darktrace’s system not only identified the threat in real-time but also provided a detailed account of the user’s actions, which were contrary to their usual behaviour patterns. This incident underscores the efficacy of ML in identifying subtle, insider threats that typically evade traditional security measures.
Case Study 2: SparkCognition and DeepArmor
SparkCognition, an AI company established in 2013, developed DeepArmor, an endpoint protection platform that employs machine learning to secure devices against malware attacks. DeepArmor is designed to offer more robust protection than traditional, signature-based antivirus programs by using machine learning to identify and block known and unknown malware strains.
Real-world Application: In a notable demonstration of its capabilities, DeepArmor was subjected to a range of malware samples, including the infamous WannaCry ransomware. Traditional, signature-based systems failed to identify the ransomware because it was a zero-day attack –malware that hadn’t been seen before. In contrast, DeepArmor identified and quarantined the ransomware based on its behaviour and code patterns. This instance highlights how AI-driven security solutions offer superior protection by identifying malware based on attributes beyond mere signatures.
Case Study 3: Mastercard and Decision Intelligence
Mastercard, a leader in global payments, has integrated AI into its security with a platform called Decision Intelligence. This system uses machine learning to analyze transaction details, such as customer habits, to make more informed decisions about whether transactions are legitimate or potentially fraudulent.
Real-world Impact: One of the issues in digital transaction processes is false declines – legitimate transactions that are declined due to suspected fraud. For retailers and customers alike, this is a source of frustration and lost revenue. Mastercard’s Decision Intelligence has significantly reduced these incidents. In one scenario, a customer made several high-value purchases in a short period, which triggered fraud alerts with traditional systems. However, Decision Intelligence, recognizing the customer’s consistent location and using the purchasing history, allowed the transactions. This example demonstrates how AI can lead to more nuanced decision-making, reducing false positives, and improving the customer experience.
These case studies illustrate the practical benefits and real-world impact of AI and ML in cybersecurity. They highlight not only the defensive capabilities of these technologies but also their potential to enhance operational efficiency and user experience.
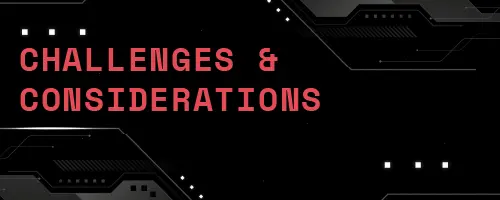
While AI and ML are transforming cybersecurity, their implementation is not without challenges. Businesses need to consider several factors to effectively integrate these technologies into their cybersecurity strategies:
- Data Privacy and Ethical Concerns: AI and ML systems require access to vast amounts of data, which often includes sensitive information. Ensuring this data is collected, processed, and stored in compliance with privacy laws and ethical standards is a significant challenge. Businesses must navigate a complex legal landscape and may need to implement additional measures to protect data privacy and comply with regulations like the General Data Protection Regulation (GDPR).
- Quality of Data: The effectiveness of AI and ML models depends on the quality of the data they are trained on. Inaccurate, outdated, or biased data can lead to incorrect conclusions and vulnerabilities. Ensuring data integrity requires robust data validation processes and ongoing data management efforts.
- Over-reliance on Automation: While AI and ML significantly enhance efficiency, an over-reliance on automated systems can be risky. These technologies can sometimes produce false positives or negatives, and their decision-making processes can be opaque, leading to the so-called “black box” problem. Human oversight is necessary to review and interpret AI and ML findings, especially when they inform critical decisions.
- Security of AI Systems Themselves: AI-driven cybersecurity solutions also present new targets for attackers. Threat actors may attempt to corrupt the data used to train ML models, a strategy known as a “poisoning attack,” or exploit vulnerabilities in the AI software itself. Protecting these systems requires a cybersecurity strategy that considers the unique vulnerabilities of AI and ML.
- Skill Gaps and Resource Constraints: Implementing and managing AI and ML solutions require specialized skills that may be scarce in many organizations. Additionally, these technologies can be resource-intensive, requiring significant computing power and specialized hardware. Businesses must consider these resource requirements and potentially invest in upskilling their teams or partnering with specialized vendors.
- Evolving Cyber Threats: Cybercriminals are also leveraging AI and ML to develop more sophisticated attack methods. For instance, they may use ML to identify new vulnerabilities or automate attacks on a scale previously impossible. Businesses must stay informed about these evolving threats and continuously update their cybersecurity strategies.
- Regulatory and Compliance Challenges: As AI and ML technologies advance, so does the regulatory landscape governing their use. Compliance with emerging standards and regulations requires businesses to be agile and adaptable, often necessitating regular audits and updates to their systems and practices.
AI and ML stand as twin pillars in modern cybersecurity frameworks, offering a blend of versatility, efficiency, and predictive prowess.
Conclusion
AI and ML are not just buzzwords; they are powerful tools that are reshaping the cybersecurity landscape. As cyber threats grow in complexity, the proactive and dynamic defence capabilities offered by AI and ML will be indispensable for businesses worldwide. By understanding their potential and integrating them effectively, businesses can safeguard their assets and foster trust among their stakeholders.
Cited Resources: Darktrace Enterprise Immune System | SparkCognition’s DeepArmor | Mastercard’s Decision Intelligence